Introduction
In today’s digital era, the significance of data in guiding business decisions cannot be overstated. Whether you’re steering a large corporation or a small start-up, data-driven decision making offers a pathway to more informed, objective, and ultimately successful outcomes. But what exactly does it mean to be data-driven, and how can organisations effectively implement this approach? In this blog, the &Element team explore the best practices and techniques to make data-driven decision making a cornerstone of your business strategy.
Blog Overview:
- Introduction
- What is Data-Driven Decision Making?
- Best Practices for Data-Driven Decision Making
- Techniques for Effective Data-Driven Decision Making
- Examples of Data Driven Decision Making
- Conclusion
What is Data-Driven Decision Making?
Data-driven Decision Making involves basing your decisions on data analysis rather than intuition or observation alone.
It requires gathering relevant data, interpreting it correctly, and using the insights gained to guide strategic choices. By leveraging data, businesses can reduce uncertainty, uncover new opportunities, and achieve a competitive edge.
Why is Data-Driven Decision Making Important?
In a landscape where businesses generate and have access to vast amounts of data, the ability to make informed decisions is more crucial than ever. Here are some reasons why DDDM is essential:
- Enhanced Accuracy: Data provides concrete evidence, reducing the reliance on guesswork.
- **Improved Efficiency: **Data analysis can streamline operations by identifying what works and what doesn’t.
- Better Forecasting: Predictive analytics allows businesses to anticipate trends and plan accordingly.
- **Increased Accountability: **Decisions backed by data are easier to justify to stakeholders.
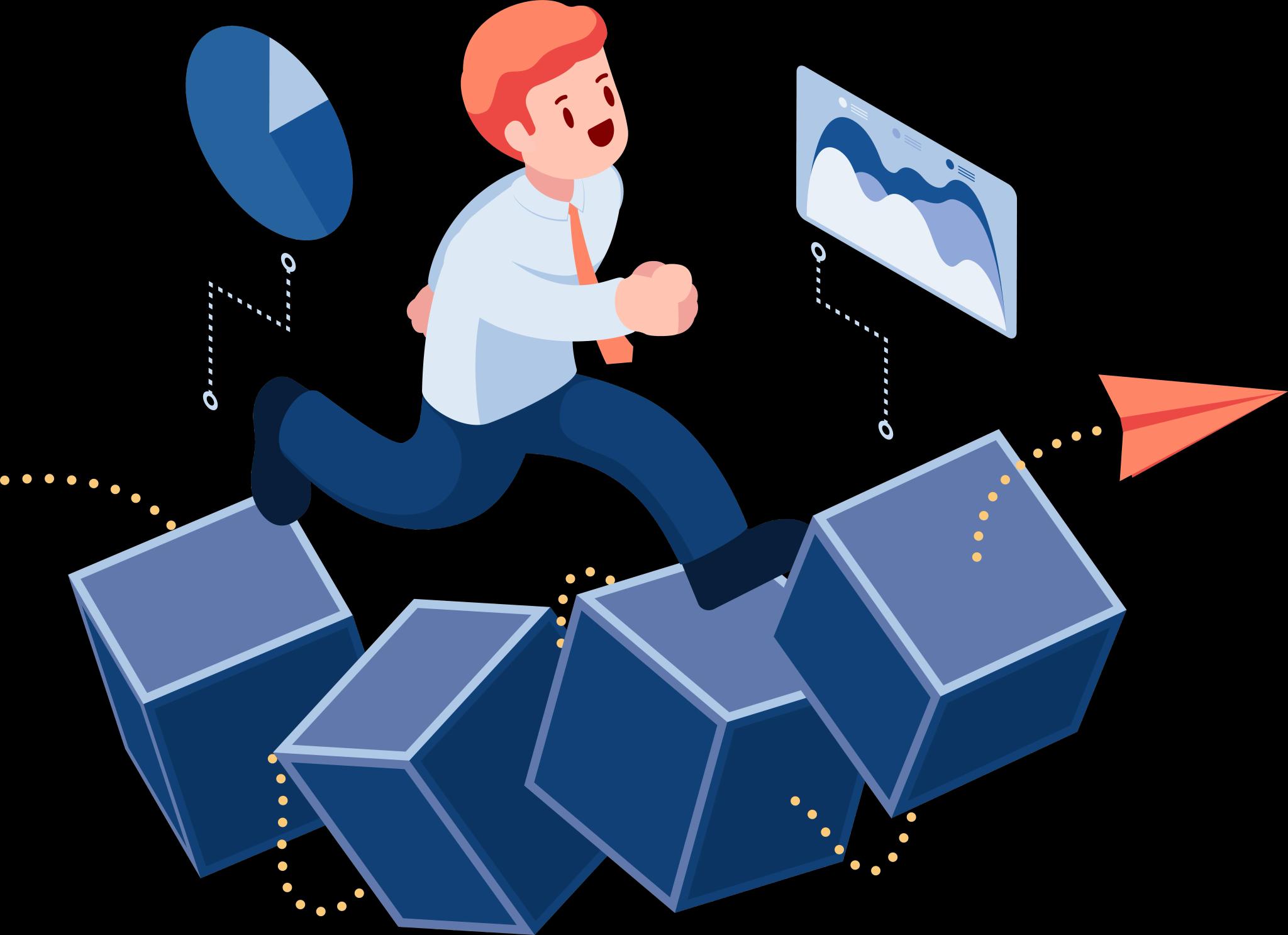
Best Practices for Data-Driven Decision Making
1. Define Clear Objectives
Before diving into data, it’s crucial to establish clear objectives. Understand what you want to achieve and what questions you need to answer. This will guide your data collection and analysis efforts, ensuring they are aligned with your business goals.
2. Ensure Data Quality
The quality of your decisions is only as good as the data you base them on. Invest in tools and processes that ensure your data is accurate, up-to-date, and relevant. Cleanse your data regularly to remove inaccuracies and inconsistencies.
3. Use the Right Tools and Technologies
The right tools are vital for effective data analysis. Whether it’s data visualisation software, machine learning algorithms, or statistical analysis tools, ensure that your team has access to the technology that best suits your needs. Familiarise yourself with platforms like Tableau, Power BI, or Python’s data analysis libraries, which can offer robust solutions for your data needs.
4. Cultivate a Data-Driven Culture
Encouraging a culture that values data-driven decision making is key. This involves training employees to understand the importance of data, how to interpret it, and how to use it in their decision-making processes. Leadership should lead by example, demonstrating a commitment to using data in strategic planning.
5. Prioritise Data Security
With great data comes great responsibility. Ensure that your data is protected against breaches and unauthorised access. This involves implementing strong cybersecurity measures, encrypting sensitive information, and complying with data protection regulations like GDPR.
6. Integrate Data Across Departments
Data silos can hinder the effectiveness of your decision-making. Integrate data across departments to ensure a holistic view of the business. This can lead to more comprehensive insights and better-aligned strategies.
7. Regularly Review and Adjust
Data-driven decision making is not a one-off process. Regularly review your data, assess the outcomes of your decisions, and be prepared to adjust your strategies as new data becomes available. This agility can help your business stay ahead in a fast-paced environment.
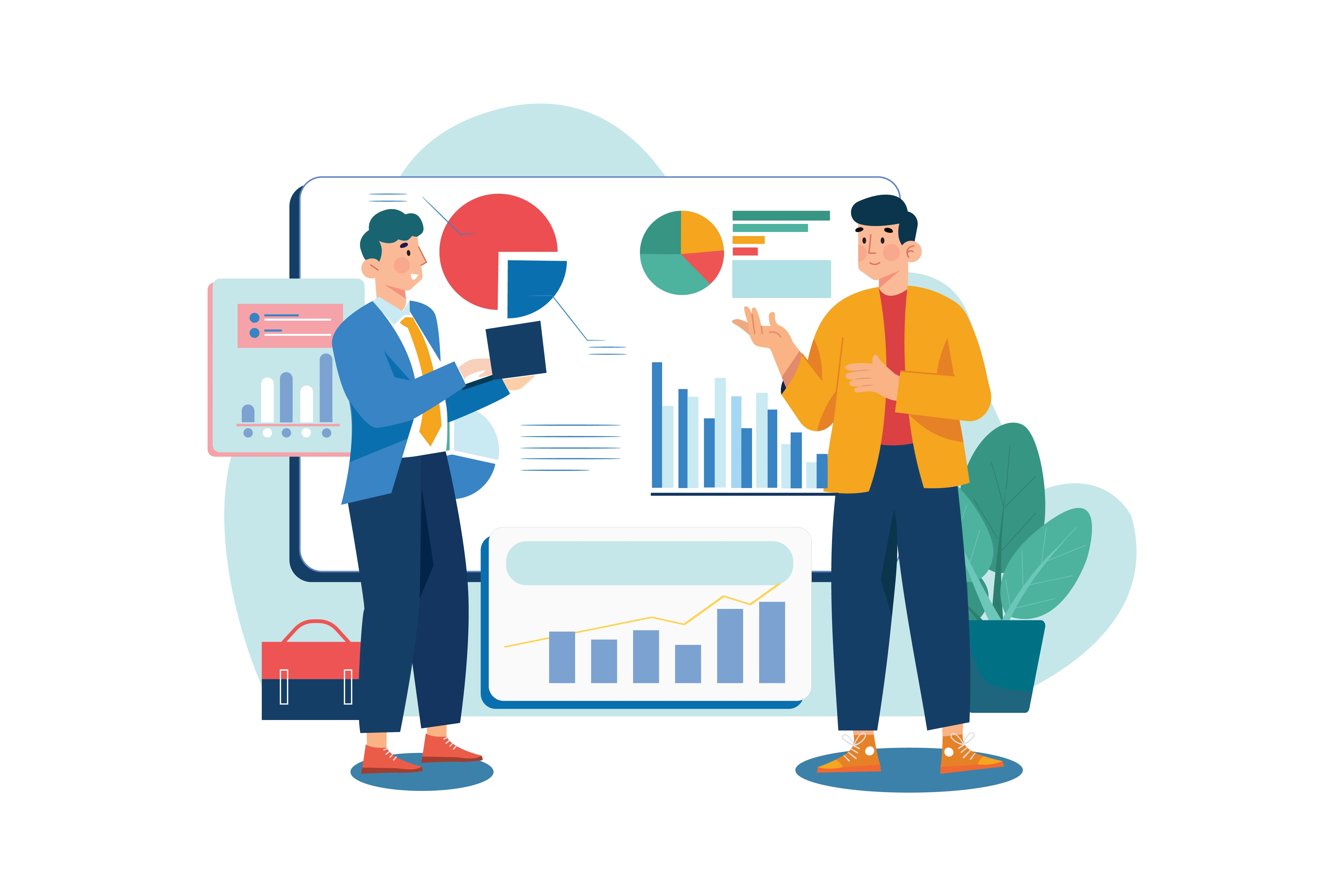
Techniques for Effective Data-Driven Decision Making
1. Predictive Analytics
Predictive analytics involves using historical data to predict future outcomes. By identifying patterns and trends, businesses can make more informed decisions about future strategies. For instance, retailers can forecast sales trends, and finance teams can predict cash flow issues before they arise.
2. A/B Testing
A/B testing, or split testing, allows businesses to compare two versions of a variable (like a web page or a marketing email) to determine which performs better. This technique provides concrete evidence of what works, enabling you to make data-backed decisions about changes to your products, services, or marketing strategies.
3. Data Visualisation
Data visualisation turns complex data sets into easily understandable visual formats, such as charts, graphs, and heat maps. This technique helps decision-makers quickly grasp insights and trends, making data more accessible and actionable.
4. Machine Learning
Machine learning algorithms can process large data sets and identify patterns that humans might miss. These algorithms can be used to automate decision-making processes, such as credit scoring, fraud detection, or personalised marketing campaigns.
5. Customer Segmentation
By analysing customer data, businesses can segment their audience into different groups based on behaviours, preferences, or demographics. This allows for more targeted marketing, personalised customer experiences, and improved customer retention strategies.
Examples of Data Driven Decision Making
Example | Outcome |
A large retail chain collects data on customers' purchasing habits, preferences, and demographics. By analysing this data, they segment customers into different groups and create personalised marketing campaigns tailored to each segment. For instance, customers who frequently buy baby products might receive targeted promotions for baby food or diapers. | The retailer can increase sales by offering relevant promotions to the right customers, improving customer satisfaction and loyalty. |
A hospital system uses data analytics to predict which patients are at higher risk of readmission after being discharged. By analysing historical data such as patient demographics, medical history, and treatment plans, the hospital can identify trends and factors that contribute to readmission. | The hospital can implement targeted interventions for at-risk patients, such as follow-up care or additional resources, leading to improved patient outcomes and reduced readmission rates. |
A bank utilises machine learning algorithms to analyse transaction data in real-time, identifying patterns that might indicate fraudulent activity. For example, if a customer's credit card is used in two different countries within a short time frame, the system flags it as suspicious. | By promptly identifying and stopping fraudulent transactions, the bank protects its customers' assets and reduces financial losses. |
Conclusion
Data-driven decision making is no longer a luxury but a necessity in today’s competitive business environment. By adopting the best practices and techniques outlined above, organisations can harness the power of data to drive better outcomes. Remember, the journey to becoming a data-driven organisation is continuous, requiring constant learning, adaptation, and a commitment to leveraging data at every level of the decision-making process.
Consider integrating &Elements into your strategy to enhance your ability to make data-driven decisions effectively. By doing so, you can ensure that your data is not only insightful but also actionable, allowing your business to thrive in the data-driven world.